Integrating Time Series Analysis to Enhance Demand Forecasting
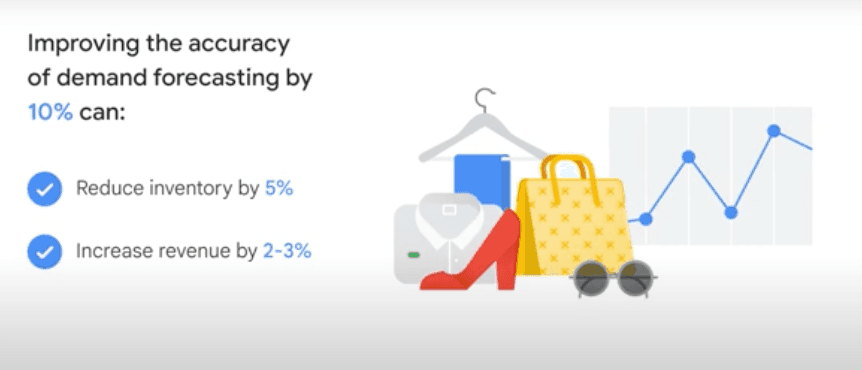
Published on 11/13/2024 by Joseph Ani
Introduction
Time series analysis is a technique used to predict future values based on past observations. The application of inventory management systems drawing insights from product advertisements, product attributes, customer reviews, locations and other factors.
Understanding Time Series Forecasting
A sequence of data points collected at regular intervals over a period of time, that is used to identify patterns and trends in the data which can be used to make predictions about future values. Time series exists in many fields such as economics, retail sales, heart rate monitoring, restaurant peak hours, weather forecasting, traffic, call center demand and stock market analysis.
The types of time series forecasting
1. Univariate: A single variable like historical sales data. Seasonal patterns or spikes in demand for certain products or the cyclical trends of everyday items are analyzed in this type of forecasting.
2. Multivariate: This refers to Multiple factors affecting the demand for a product. This shows how various factors such as price, promotion, advertising interact and influence each other. For example, a cold winter season might increase the demand for woolen clothes, but a concurrent increase in the price of wool might reduce the demand for woolen clothes.
3. Covariate: This is a dynamic time series forecasting that influences demand from external factors. Examples include weather patterns, holidays, economic indicators, marketing campaigns, competittor pricing and social media buzz. These factors can be incoporated into its forecasting model to provide a more nuanced prediction of future demand.
4. Attributes: Are static features, where AI.ML models goes beyond the data itself. It analyzes attributes like brand, color, size, price, customer reviews to predict how these elements contribute to demand.
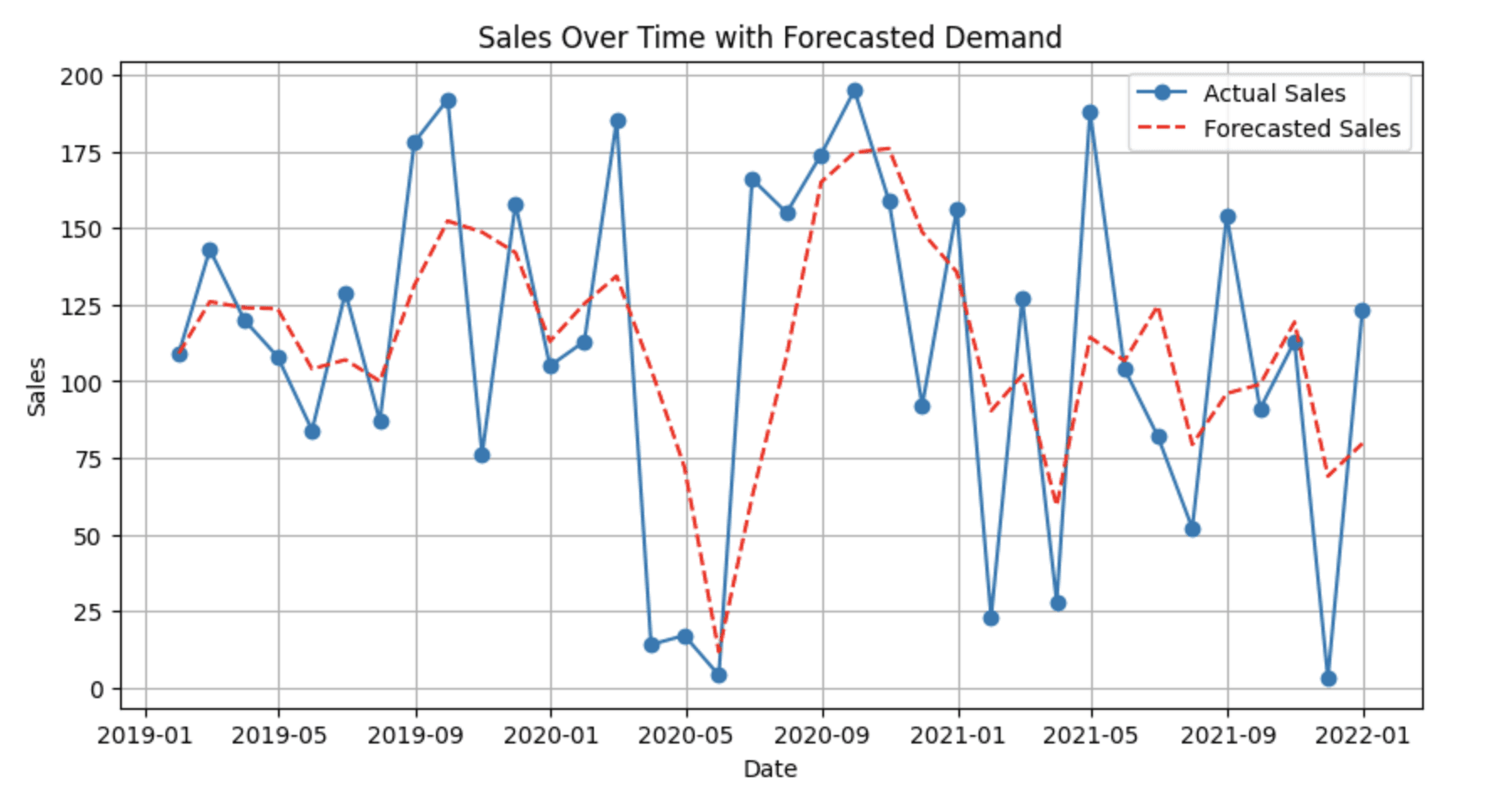
Conclusion
By integrating time series analysis into their forecasting models, e-commerce businesses can enhance their demand forecasting capabilities, leading to more accurate inventory management, optimized supply chain logistics, and improved customer satisfaction.